Page History: Yang Yu @ NJUCS
Compare Page Revisions
Page Revision: 2012/08/23 23:20
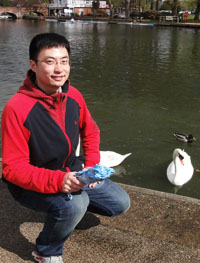 | 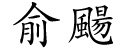 Yang Yu (Y. Yu) Can be pronounced as "young you"
Ph.D., Assistant Researcher LAMDA Group, Department of Computer Science, Nanjing University
Office: 919, Computer Science Building, Xianlin Campus
email: yuy@nju.edu.cn (alternatives: yuy@lamda.nju.edu.cn, eyounx@gmail.com) | 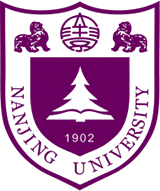 |
I received my Ph.D. degree in Computer Science from Nanjing University in 2011 (supervisor Prof.
Zhi-Hua Zhou), and then joined the Department of Computer Science and Technology of Nanjing University as an assistant researcher in 2011.
I am interested in artificial intelligence, particularly, machine learning, evolutionary computation and robotics. I have published papers in international conferences (e.g. IJCAI) and journals (e.g. Artificial Intelligence), and won several awards such as Grand Champion (with other LAMDA members) of PAKDD'06 Data Mining Competition, the best paper award of PAKDD'08, the best theory paper award of GECCO'11, and China Computer Federation Outstanding Doctoral Dissertation Award in 2011.
(
Detailed CV)
Research
I am interested in
artificial intelligence including subfields of
machine learning and
evolutionary computation, especially,
Selected work: (
full publication list)
- General approaches to running time analysis of metaheuristic optimization algorithms: (with Chao Qian and Zhi-Hua Zhou)
The running time, or the computational complexity, of evolutionary algorithms and other metaheuristic algorithms is one of the most important theoretical issue to understand these algorithms.- We developed the convergence-based approach (PDF-aij08) that can be applied to obtain running time bounds of a large range of metaheuristic algorithms.
- By comparing two algorithms, We developed a new analysis tool, switch analysis (tr11|PDF-ppsn10) that can lead to tighter running time bounds.
- Using switch analysis to compare problem instances, we can identify the hardest and the easiest problem instances for a metaheuristic algorithm in a problem class (PDF-ppsn12).
- Approximation ability of evolutionary optimization: (with Xin Yao and Zhi-Hua Zhou)
Evolutionary algorithms are most commonly used to obtain good-enough solutions rather than optimal solutions in practice, which relates to their approximation ability. We developed the SEIP framework (PDF-aij12) to characterize the approximation ability of evolutionary algorithms, and showed that they can achieve the currently best-achievable approximation ratio for the k-set cover problem, which also reveals the advantage of evolutionary algorithms over the well known greedy algorithm.
- The role of diversity in ensemble learning (with Nan Li, Yu-Feng Li and Zhi-Hua Zhou)
Ensemble learning is a machine learning paradigm that achieves the state-of-the-art performance. Diversity was believed to be a key to a good performance of an ensemble approach, which, however, previously servered only as a heuristic idea.- By the diversity regularized machine (PDF-ijcai11), we showed that diversity plays a role of regularization as in popular statistical learning approaches.
- We proved that diversity defined on hypothesis output space plays a role of regularization, and proposed the diversity regularized ensemble pruning to prune Bagging classifiers. (PDF-ecml12)
(
My Goolge Scholar Citations)
Recent Professional Activities
Teaching
- Data Mining. (for M.Sc. students. Fall, 2012) >>>Course Page>>>
- Introduction to Data Mining. (for undergraduate students. Spring, 2012) Teaching Assistant Page
- (Assistant) Data mining. (for graduate students. Fall, 2007) Teaching Assistant Page
- (Assistant) Algorithm Design and Analysis. (for undergraduate students. Fall, 2005)
Mail: National Key Laboratory for Novel Software Technology, Nanjing University, Xianlin Campus Mailbox 603, 163 Xianlin Avenue, Qixia District, Nanjing 210046, China