Invited
speakers
Prof.
Bin Yu
(UC Berkeley, USA)
Stability and Large
Scale Statistical Inference (Slides)
Abstract
Information technolgoy has enabled collection of
large amounts of and high-dimensional data across fields in science,
engineering, social science, commerce, and beyond. Reproducibility is
imperative for any meaningful discovery from these data. Statistical
machine learning analyses are often used to bring the discoveries and,
as a minimal manifestitation of reproducibility, conclusions from
statistical analyses have to be stable or robust to "reasonable"
perturbations to data and to the model used. Examples of data
perturbation schemes include Jacknife, bootstrap, and cross-validation
while robust statistics aims at studying perturbations to models. In
this talk, the indispensable requirement of stability is advocated for
large scale statistical inference. A movie-reconstruction from fMRI
data motivates the need for stablity, and a new estimation stability
revision on CV for Lasso is proposed to lead to a much simpler and more
reliable model. Finally, a novel stability analytical argument is seen
to drive new results that shed light on the intriguing interactions
between sample to sample varibility and heavier tail error distribution
(e.g. double-exponential) in high-dim robust statistics.
Bio
Bin Yu is
Chancellor's Professor in the Departments of Statistics and of
Electrical Engineering & Computer Science at UC Berkeley. She has
published over 100 scientific papers in premier journals in Statistics,
EECS, remote sensing and neuroscience, in a wide range of research
areas including empirical process theory, information theory (MDL),
MCMC methods, signal processing, machine learning, high dimensional
data inference (boosting and Lasso and sparse modeling in general), and
interdisciplinary data problems. She has served on many editorial
boards for journals such as Annals of Statistics, Journal of American
Statistical Association, and Journal of Machine Learning Research.
She was a 2006 Guggenheim Fellow, co-recipient of the Best Paper Award
of IEEE Signal Processing Society in 2006, and the 2012 Tukey Memorial
Lecturer of the Bernoulli Society (selected every four years). She is a
Fellow of AAAS, IEEE, IMS (Institute of Mathematical Statistics) and
ASA (American Statistical Association).
She is currently President-Elect of IMS (Institute of Mathematical
Statistics). She is serving on the Scientific Advisory Board of IPAM
(Institute ofr Pure and Applied Mathematics) and on the Board of
Mathematical Sciences and Applications of NAS. She was co-chair of the
National Scientific Committee of SAMSI (Statistical and Applied
Mathematical Sciences Institute), and on the Board of Governors of
IEEE-IT Society.
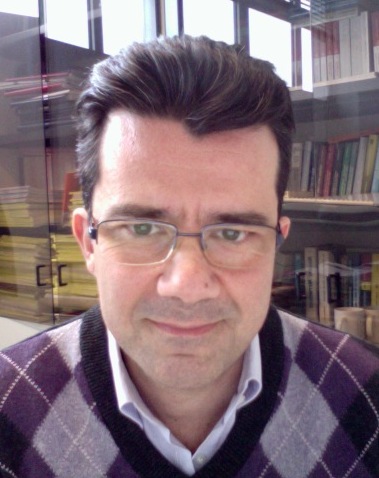
Prof.
Marcello
Pelillo (Universitą Ca' Foscari Venezia, Italy)
(Marcello
has to cancel the trip due to unexpected family reasons.)
Similarity-Based
Pattern Recognition: A Game-Theoretic Perspective
Abstract [Read the full extended
abstract]
The
classical approach to deal with non-geometric (dis)similarities is "em-
bedding", which refers to any procedure that takes a set of
(dis)similarities as input and produces a vectorial representation of
the data as output, such that the proximities are either locally or
globally preserved. This is an (approximate or ideal) isometric mapping
which finds a set of vectors in an instance-specific Euclidean space
that are capable of describing the data satisfactorily. Embedding
approaches are all based on the assumption that the non-geometricity of
similarity information can be eliminated or some-how approximated away.
When this is not the case, i.e., when there is significant information
content in the non-geometricity of the data, however, alternative
approaches are needed.
In this talk, I will maintain that game theory offers an elegant and
powerful conceptual framework which serves well this purpose. The
development of game theory in the early 1940's by von Neumann was a
reaction against the then dominant view that problems in economic
theory can be formulated using standard methods from optimization
theory. Indeed, most real-world economic problems typically involve
conflicting interactions among decision-making agents that cannot be
adequately captured by a single (global) objective function, thereby
requiring a different, more sophisticated treatment. Accordingly, the
main point made by game theorists is to shift the emphasis from
optimality criteria to equilibrium conditions, namely to the search of
a balance among multiple interacting forces. Interestingly, the
development of evolutionary game theory in the late 1970's by Maynard
Smith offered a dynamical systems perspective to game theory, an
element which was totally missing in the traditional formulation, and
provided powerful tools to deal with the equilibrium selection problem.
As it provides an abstract theoretically-founded framework to elegantly
model complex scenarios, game theory has found a variety of
applications not only in economics and, more generally, in the social
sciences but also in different fields of engineering and information
technologies. In the talk, I will describe recent attempts aimed at
formulating (or interpreting) several pattern recognition and machine
learning problems from a game-theoretic perspective. Indeed, many
problems within these fields can naturally be formulated at an abstract
level in terms of a game where (pure) strategies correspond to class
labels and the payoff function is expressed in terms of competition
between the hypotheses of class membership. In particular, I will focus
on data clustering, semi-supervised learning, structural matching, and
contextual pattern recognition. Further, I will discuss potential
applications of game theory within the context of multiple classifier
systems.
Bio
Marcello
Pelillo joined the faculty of the University of Bari, Italy, as an
Assistant professor of computer science in 1991. Since 1995, he has
been with the University of Venice, Italy, where he is currently a Full
Professor of Computer Science.
He leads the
Computer Vision and Pattern Recognition Group and has served from 2004
to 2010 as the Chair of the board of studies of the Computer Science
School. He held visiting research positions at Yale University, the
University College London, McGill University, the University of Vienna,
York University (UK), and the National ICT Australia (NICTA). He has
published more than 130 technical papers in refereed journals,
handbooks, and conference proceedings in the areas of computer vision,
pattern recognition and neural computation.
He serves
(or has served) on the editorial board for the journals IEEE
Transactions on Pattern Analysis and Machine Intelligence and Pattern
Recognition, and is regularly on the program committees of the major
international conferences and workshops of his fields. In 1997, he
co-established a new series of international conferences devoted to
energy minimization methods in computer vision and pattern recognition
(EMMCVPR) which has now reached the ninth edition. He is (or has been)
scientific coordinator of several research projects, including SIMBAD,
an EU-FP7 project devoted to similarity-based pattern analysis and
recognition. Prof. Pelillo is a Fellow of the IEEE and of the IAPR.
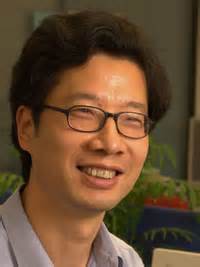
Prof. Xin Yao (University of Birmingham, U.K.)
From
Evolutionary Computation to Ensemble Learning (Slides)
Abstract
Designing a monolithic system for a
large and complex learning task is hard. Divide-and-conquer is a common
strategy in tackling such large and complex problems. Artificial
speciation and niching have long been used in evolutionary computation
as one way towards automatic diivide-and-conquer. It turns out that
several key ideas in speciated evolutionary algorithms are closely
linked to ensemble learning in general, especially diversity in
ensembles. This talk reviews selected work on this topic and
illustrates the link between evolutionary computation and ensemble
learning using the example of negative correlation learning. Then the
importance of diversity is demonstrated using examples from online
learning and class imbalance learning. As a practical solution towards
accurate and diverse ensembles, multi-objective ensemble learning is
advocated. Finally, a recent application of multi-objective learning to
software effort estimation will be described.
Xin Yao is a professor of computer science from the University of
Birmingham, UK. He was also a Distinguished Visiting Professor of the
University of Science and Technology of China (USTC), P. R. China, and
a visiting professor of three other universities. He is a Fellow of the
IEEE, Editor-in-Chief (2003-08) of IEEE Transactions on Evolutionary
Computation, an associate editor or an editorial board member of 11
other journals. He was the winner of 2001 IEEE Donald G. Fink prize
paper award and several other best paper awards. His research interests
include evolutionary computation, neural network ensembles, and their
applications. He has more than 300 refereed publications in those
areas. He is currently the Director of the Centre of Excellence for
Research in Computational Intelligence and Applications (CERCIA) ,
which is focused on applied research and knowledge transfer to industry.
References:
• X. Yao and Y. Liu, ``Making use of population information in
evolutionary artificial neural networks,'' IEEE Transactions on
Systems, Man and Cybernetics, Part B: Cybernetics, 28(3):417-425, June
1998.
• A Chandra and X. Yao, ``Ensemble learning using multi-objective
evolutionary algorithms,'' Journal of Mathematical Modelling and
Algorithms, 5(4):417-445, December 2006.
• L. L. Minku and X. Yao, "DDD: A New Ensemble Approach For Dealing
With Concept Drift,'' IEEE Transactions on Knowledge and Data
Engineering, 24(4):619-633, April 2012.
• S. Wang and X. Yao, ``Multi-Class Imbalance Problems: Analysis and
Potential Solutions,'' IEEE Transactions on Systems, Man and
Cybernetics, Part B, 42(4):1119-1130, August 2012.
• L. L. Minku and X. Yao, ``Software Effort Estimation as a
Multi-objective Learning Problem,'' ACM Transactions on Software
Engineering and Methodology, to appear.
Bio
Xin Yao is a
professor of computer science from the University of
Birmingham, UK. He was also a Distinguished Visiting Professor of the
University of Science and Technology of China (USTC), P. R. China, and
a visiting professor of three other universities. He is a Fellow of the
IEEE, Editor-in-Chief (2003-08) of IEEE Transactions on Evolutionary
Computation, an associate editor or an editorial board member of 11
other journals. He was the winner of 2001 IEEE Donald G. Fink prize
paper award and several other best paper awards. His research interests
include evolutionary computation, neural network ensembles, and their
applications. He has more than 300 refereed publications in those
areas. He is currently the Director of the Centre of Excellence for
Research in Computational Intelligence and Applications (CERCIA) ,
which is focused on applied research and knowledge transfer to
industry.
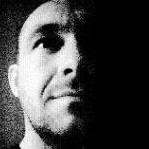
Prof.
Marco Loog (Delft University of Technology, The Netherlands;
University of Copenhagen, Denmark)
Semi-Supervised
Learning: Dipping, Loss, Constraints, and the General Interest in Basic
Research Questions (Slides)
Abstract
I will provide a coarse overview of the principal
approaches to
semi-supervised learning and pass in review my own work in that same
field. The latter runs counter to the mainstream and provides a rather
different take on semi-supervised learning. Next to introducing the
basic tenet in my reserach, I will roughly sketch the main approach I
have been studying, provide some simple instantiations of it, and
discuss the main challenges ahead.
Bio
Marco Loog
received an
M.Sc. degree in mathematics from Utrecht University and in 2004 a Ph.D.
degree from the Image Sciences Institute for the development and
improvement of contextual statistical pattern recognition methods and
their use in the processing and analysis of images. Since 2008, Marco
resides at Delft University of Technology where he works as an
assistant professor in the Pattern Recognition Laboratory. He currently
is chair of TC1 of the IAPR, holds a bunch of associate editorships, is
honorary full professor in pattern recognition at the University of
Copenhagen, and is also affiliated to Eindhoven University of
Technology. Marco's research interests include multiscale image
analysis, semi-supervised and multiple instance learning, saliency,
computational perception, the dissimilarity approach, and black math.
|